Data Quality: The Keystone of Effective AI Implementation
Everyone wants to dive straight into the most important and exciting thing to happen to computing since the internet: AI. It’s a catch phrase which the media has been pounding into our heads for a few years now. “AI: GOOD!” and “AI: BAD!!!” posts on news websites have become the de facto “hotness” in reporting.
AI is not inherently good or evil. This technology does not meet the true definition of AI, a full self-aware system; artificial intelligence in the current context is just a system of data collection and analysis, nothing else. Today's AI is not capable of autonomously judging humans as a lower species and triggering the end of the world. It would be more accurate to call the technology an "expert system", though it's not as catchy as "AI".
AI is undeniably useful, though. These products work in conjunction with data collection systems and emulate the decision-making ability of a like-minded human expert. Some are generic in their scope (such as ChatGPT), others are specific to a company’s product line (like AI help bots on a support site for an accounting service). What separates one AI system from another is the data it has access to, and how the decision-making process is emulated.
Let’s talk about AI data use.
To help AI learn and make decisions, it needs data—lots of it. This is often sourced from online interactions, sensors/devices, public records and databases, or from specific business operations.
Every time you click on a website, make a purchase, or even ‘like’ a post on social media, you generate data. Companies use this data to teach AI about shopping patterns, popular products, or trending topics.
Devices like smartphones, fitness trackers, and smart home devices collect data all the time. This can include everything from your location and how many steps you take, to the temperature in your living room. “They’re watching you” is an apt insight, but it’s not meant to be malicious. In almost every occurrence, you control what’s being sent to these data collectors.
Information like weather reports, economic indicators, or transportation schedules that are publicly available can also be used to feed AI. This helps AI understand patterns or trends in larger systems like climate or traffic.
Within a company, AI can learn from data like sales records, inventory levels, or customer service interactions. This helps businesses predict trends, manage stock, or improve customer satisfaction.
How exactly is the data used by AI?
Once AI has access to data, it uses algorithms to find patterns and insights. An algorithm is just a set of instructions telling the AI what to do with the data.
First, AI analyzes the data to learn about patterns and relationships. For example, it might learn that when it rains, more people buy umbrellas.
Based on what it has learned, AI can then make predictions or decisions. If the weather forecast says it will rain tomorrow, AI might suggest stocking more umbrellas near cashiers at a grocery store.
“Good… Bad… I’m the guy with the umbrella.”
For AI to be effective, it needs high-quality data—data that is accurate, comprehensive, and relevant. Poor quality data can lead to incorrect predictions, just like studying from an outdated textbook can lead to failing a test.
The quality of data directly impacts how well AI systems can perform their tasks. Good data leads to more reliable and accurate AI outputs, while bad data can cause AI systems to make incorrect predictions, leading to faulty decisions and potentially significant consequences, especially in critical applications like healthcare or finance.
Unfortunately, there is currently no measurement we can easily use to rate the quality of an AI’s data. That will likely come in the future, though it is needed now.
Safeguarding your AI use by asking about the data
You, your company, as an AI user, must be concerned about the data used to provide responses. Determining the quality of the data used by an AI system can be difficult, especially for users without technical expertise. However, there are several approaches and considerations that can help users assess data quality indirectly or request the necessary information from AI providers:
1. Transparency from ProvidersThe first step is to seek transparency about the data practices of the AI system provider. Users should ask providers for:
- Data Sources: Where does the data come from? Understanding the source can help gauge the reliability and relevance of the data.
- Data Collection and Processing Methods: How is the data collected and processed? Details about these methods can reveal the potential for errors or biases.
- Data Maintenance: How frequently is the data updated? This helps assess the timeliness and relevance of the data used by the AI.
Reputable AI system providers often provide documentation or reports that include:
- Data Quality Assessments: These might detail the checks and measures in place to ensure data accuracy, consistency, and completeness.
- Third-party Audits: Independent audits of the AI systems and their data practices can offer an unbiased view of the data quality and the system’s reliability.
Monitoring the performance of an AI system over time can provide indirect clues about the quality of the underlying data:
- Error Rates: High or increasing error rates might indicate issues with data quality.
- Consistency of Outputs: If the AI’s outputs are inconsistent under similar conditions, this might suggest data inconsistencies or gaps.
Gathering and analyzing feedback from users of the AI system can provide insights into its effectiveness and the possible effects of data quality:
- User Experiences: Are users consistently reporting specific issues that could be traced back to data problems?
- Comparison with Known Outcomes: For predictive systems, comparing AI predictions with actual outcomes can help assess the accuracy of the AI, which is influenced by the quality of its data.
Checking the compliance of the AI system with relevant data protection and privacy laws (like GDPR or HIPAA) can also be indicative of data quality. Compliance typically requires maintaining certain standards of data accuracy, security, and management.
Conclusion
As we increasingly use AI in our work and decision-making, it's important to remember that the real strength of AI lies in the quality of data it uses. Good data can make AI a powerful tool that helps us make better decisions and work more efficiently. Therefore, as users and leaders, we should actively ensure our AI systems are accurate and reliable. By asking for clear information, insisting on high standards for data, and regularly checking the AI tools we use, we make sure these technologies truly benefit us. Let's not just watch AI evolve; let's help shape a future where technology enhances our abilities, powered by the best data available. Remember, the better the data we give AI, the better the future we build with it.
Share this
You May Also Like
These Related Stories
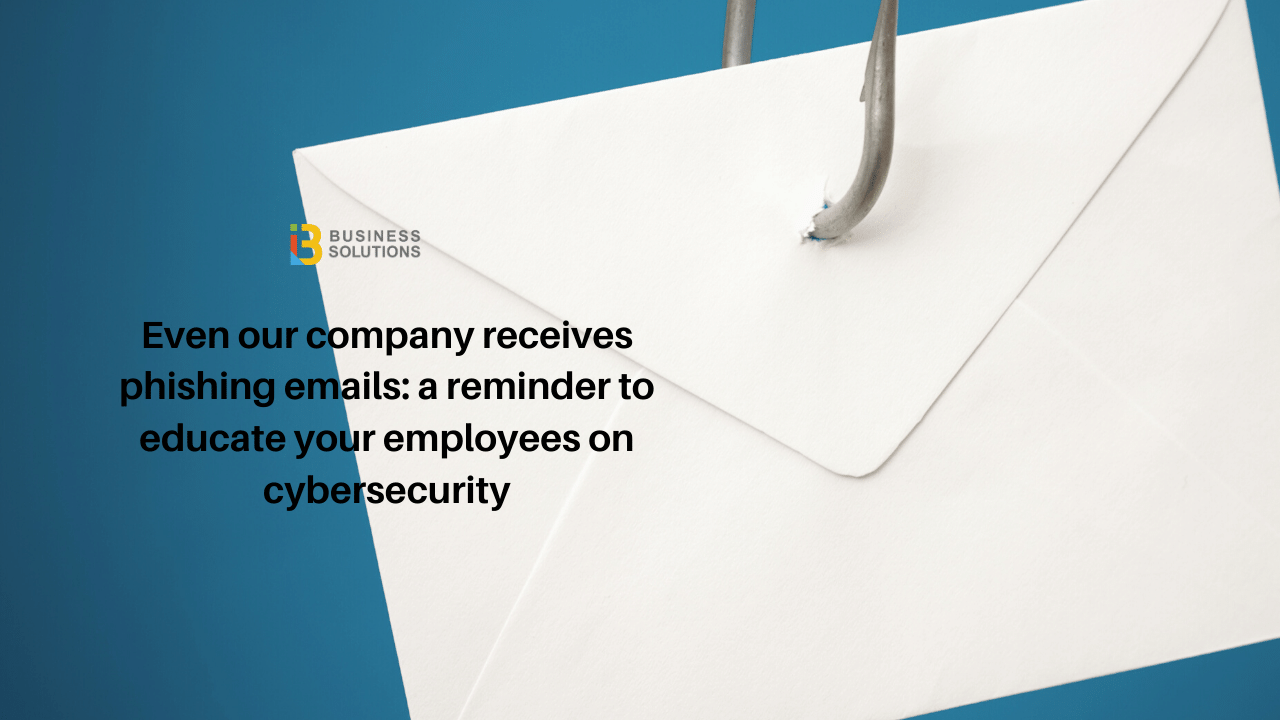
A recent Microsoft phishing email
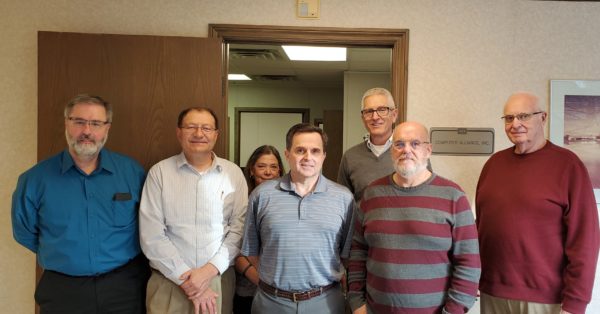
i3 Business Solutions acquires the assets of Computer Alliance Incorporated from Farmington Hills, Michigan
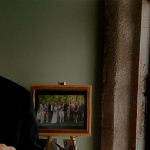
No Comments Yet
Let us know what you think